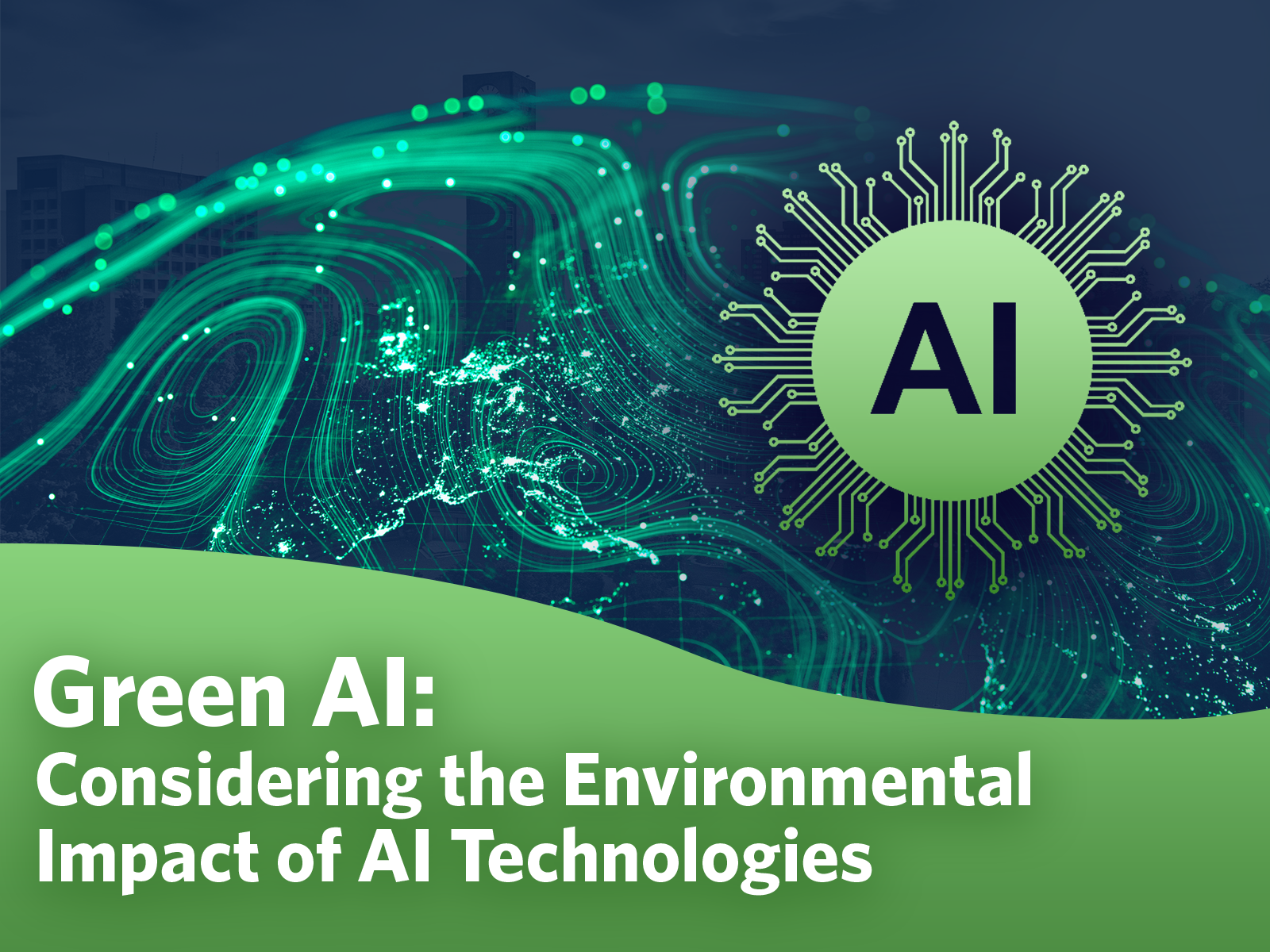
With Earth Day around the corner on April 22, 2024, UBC IT is shedding light on some of the environmental impacts of current and immerging technologies we utilize every day. This week we’re talking about Artificial Intelligence (AI).
The development of AI technologies has prioritized speed over sustainability efforts. This has resulted in a need for us—as users—to call for transparency and regulations that mandate the implementation of Green AI practices. AI technology development has largely prioritized speed by focusing on who can develop these technologies the fastest, rather than examining how they are being developed. As the dust of innovation settles, the focus of AI and sustainability must be critically evaluated.
To face this truth, we must delve into the many “how” questions surrounding AI. How are these technologies being developed? How are they being trained? How are they being used? The first hurdle in answering these questions lies in the transparency of tech companies. According to the Nature Machine Intelligence journal, “...tech companies [are] reticent about sharing data, and having no incentives to do so, any attempt at quantifying emissions remains difficult" (Dhar, Payal). A recent article in Wired magazine titled The Generative AI Race Has a Dirty Secret, emphasizes this lack of transparency, revealing that neither OpenAI nor Google have disclosed the computing cost of their AI products. Only third-party researcher analysis provides an estimate that the training of GPT-3, a model that ChatGPT is partially based on, consumes 1,287 MWh, and potentially emit more than 550 tons of carbon dioxide. Despite the openness of tech companies, the available information reveals crucial insights:
- Training AI models generate massive amounts of carbon dioxide.
- Data centres, integral to AI model training, consume large amounts of energy.
According to the recent report Sustainability Starts with the Data Center from MIT Technology Review, data centers use approximately 1.5% of total global electricity, a figure that is much higher in countries with large data storage sectors like Ireland where this figure jumps to 18%. - The operation and cooling of hardware such as GPUs, essential for AI models, contribute significantly to energy consumption (Foy, Kylie)
These insights prompt further questions; questions that researchers, including the Lincoln Laboratory, are actively addressing. In response to the environmental impacts of AI development, the concept of "green AI" has emerged, advocating for the development and deployment of AI technologies in a manner that minimizes their environmental impact. Green AI offers tangible solutions, such as optimizing the training process of AI models through energy-efficient algorithms and renewable energy sources for data centers. It also emphasizes the importance of efficient hardware design and utilization. However, despite the potential benefits of green AI, the tech industry's prevailing emphasis on speed and performance often overrides sustainability considerations.
Given that profit and speed do not inherently incentivize sustainable AI practices, it falls on governing bodies, as well as us as users and IT professionals engaging with AI technologies, to advocate for regulations that enforce more environmentally friendly development and deployment practices. By mandating transparency regarding the environmental impact of AI projects and establishing clear guidelines, we can collaborate with researchers in green AI to foster a future where the environmental impact of AI technologies is minimized.
Article by Emilyn Sim, Web Specialist Intern at UBC IT.